Quantitative Metrics for LLM Consistency Testing
Explore key metrics for evaluating LLM consistency, including self-consistency scores, semantic similarity, and contradiction detection.
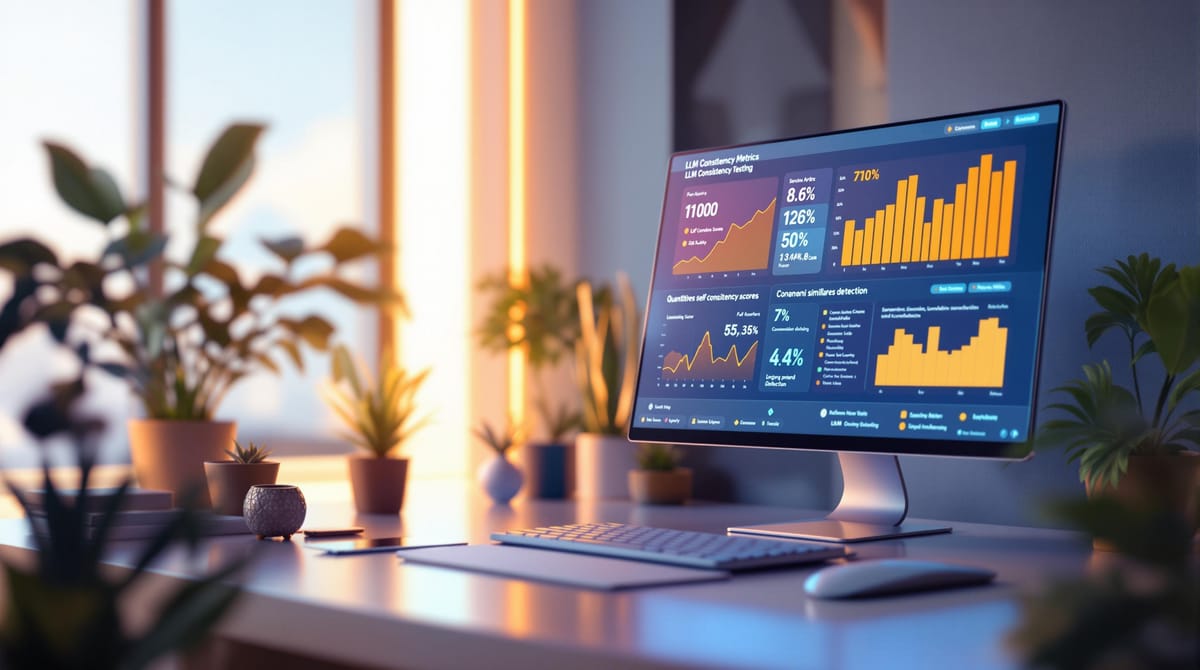
LLM consistency matters. Why? It ensures reliable responses, builds trust, and avoids risks like user frustration or compliance issues. But how do you measure it? Here are three key metrics:
- Self-Consistency Scores: Measures how often a model gives the exact same response to the same prompt.
- Semantic Similarity: Checks if responses mean the same thing, even if phrased differently.
- Contradiction Detection: Spots conflicting or logically inconsistent outputs.
Quick Comparison
Metric | Strengths | Weaknesses |
---|---|---|
Self-Consistency Scores | Simple, detects obvious errors | Misses nuanced inconsistencies |
Semantic Similarity | Captures meaning despite wording changes | May misinterpret subtle language variations |
Contradiction Detection | Identifies logical conflicts | Struggles with context-based contradictions |
Each metric offers unique insights. Use them together for a complete picture of LLM reliability.
Key Metrics for Testing LLM Consistency
Here are three main metrics used to evaluate the consistency of responses from language models. Each focuses on a different way to measure reliability.
Self-Consistency Scores
This metric checks if a language model gives the exact same answer to the same prompt when asked multiple times. It’s all about matching outputs word-for-word.
Semantic Similarity Measures
This approach looks at whether the meaning of responses is consistent, even if the wording varies. It evaluates how closely the content aligns without requiring identical phrasing.
Contradiction Detection Rates
This metric identifies how often a model provides conflicting information, either within a single response or across multiple responses. It’s especially useful for spotting logical errors.
1. Self-Consistency Scores
Self-consistency tracks how often a model produces the exact same response when given the same prompt multiple times. To calculate it, you run the same prompt through the model N times and then divide the number of identical outputs by N. A higher self-consistency score indicates the model behaves predictably, which is crucial for reducing risks in automated workflows. This metric helps establish clear, repeatable standards for consistency.
Up next, we'll look at metrics that assess how well meaning is preserved, even when wording varies.
2. Semantic Similarity Measures
Semantic similarity goes beyond exact text matching, focusing on whether different responses convey the same meaning. This method uses embedding-based comparisons - mathematical representations of text - to assess how closely responses align in intent.
A common tool for this is cosine similarity, which calculates the angular distance between response vectors. The result is a score between 0 and 1, where higher scores indicate closer alignment. Unlike strict text matching, semantic similarity allows for variations in wording as long as the underlying meaning remains consistent.
For example, imagine a customer service interaction. One response might say, "Your refund will be processed within 3-5 business days", while another states, "The refund should appear in your account by next week." Though phrased differently, semantic similarity would recognize these as conveying the same message.
BERT-based similarity scores take this a step further by analyzing contextual word meanings. This method is particularly useful for spotting subtle changes in meaning across different phrasing, user groups, or scenarios.
3. Contradiction Detection Rates
Semantic similarity helps align meaning, but contradiction detection rates reveal how often a model produces outputs that are logically inconsistent or factually conflicting.
Several factors affect these rates:
- Model architecture: More advanced models handle context better, which helps reduce inconsistencies.
- Training data: Diverse and high-quality datasets promote more consistent outputs.
- Input complexity: Complex or multi-turn prompts can increase the likelihood of contradictions.
Comparative studies on contradiction rates among large language models remain limited, highlighting an area that needs further exploration.
Metric Comparison
Here's a quick look at three key consistency metrics, along with their strengths and weaknesses:
Metric | Pros | Cons |
---|---|---|
Self-Consistency Scores | • Useful for spotting obvious errors across repeated prompts | • May overlook subtle inconsistencies in reasoning or factual details |
Semantic Similarity Measures | • Detects nuanced differences in meaning • Works well with paraphrased content |
• Can misinterpret wording variations that don't indicate actual inconsistencies |
Contradiction Detection Rates | • Great for pinpointing directly opposing statements | • Has difficulty with implicit or context-based contradictions |
Conclusion
To wrap up your testing strategy, consider these steps:
Use a combination of metrics for better insights. Latitude provides open-source tools to help you implement, track, and visualize these metrics in real-time. Choose metrics that align with your objectives, pair them strategically, monitor them regularly, and leverage Latitude's platform for automated calculations and visualizations.