Model Context Protocol: The New Standard Explained
The Model Context Protocol revolutionizes AI integration by standardizing communication, enhancing context sharing, and simplifying development.
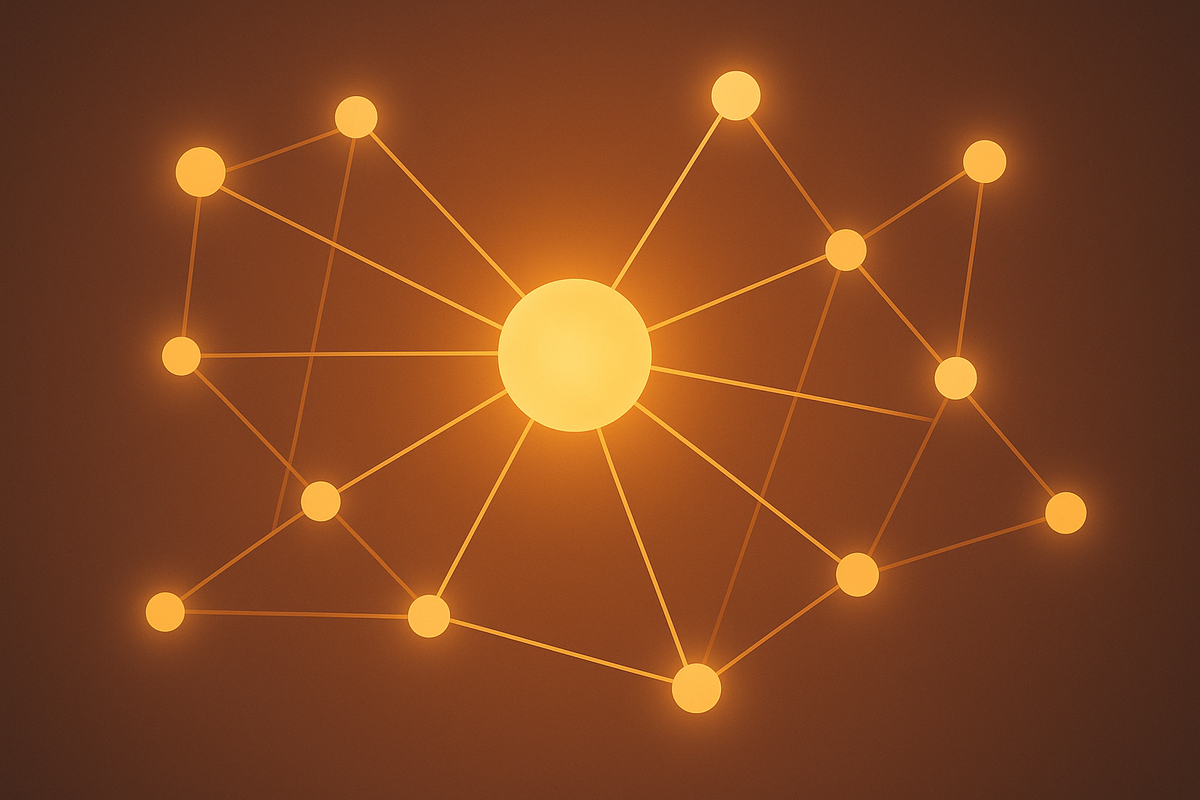
The Model Context Protocol (MCP) is a game-changer for AI integration. It acts as a universal framework that allows AI systems to communicate, share data, and maintain context seamlessly. Here's why it matters:
- Solves Fragmentation: MCP eliminates the need for custom integrations by standardizing communication.
- Maintains Context: Ensures AI systems stay aligned for accurate responses.
- Simplifies Integration: Reduces complexity with a single interface for multiple systems.
- Optimizes Performance: Dynamically manages resources and scales efficiently.
Quick Overview
- Main Features: Context persistence, dynamic scaling, state management, and advanced security.
- How It Works: A layered architecture with transport, context management, and interface layers.
- Comparison with APIs: MCP offers built-in context handling, simplified integration, and automatic updates, unlike traditional APIs.
MCP is already being adopted by leading platforms like Latitude to streamline AI development and collaboration. However, challenges like legacy system compatibility and scalability remain. Addressing these will unlock MCP's full potential to create a more connected AI ecosystem.
What is the Model Context Protocol?
The Model Context Protocol (MCP) is a system designed to streamline communication between AI modules, allowing them to share context effortlessly. This standardized method forms the basis of how MCP operates.
How MCP Works
MCP provides a structured interface that connects various AI modules. This setup allows systems to share context effectively, keeping their operations aligned and coordinated.
Main Components of MCP
MCP depends on key elements to maintain a steady flow of information. These elements simplify how different AI services integrate, ensuring smooth data exchange and shared understanding across connected systems.
MCP Technical Structure and Functions
MCP's Technical Design
MCP's design is built around a layered architecture that seamlessly integrates AI systems. This structure relies on a context-aware messaging system to synchronize states across AI modules, organized into three main layers:
- Transport Layer: Handles secure communication between components.
- Context Management Layer: Maintains a unified shared context across interactions.
- Interface Layer: Offers standardized endpoints for easier integration.
This layered system forms the backbone of MCP's core functionality.
Key MCP Features
Using this architecture, MCP enables efficient and reliable interactions in AI systems. Here's how its features work:
Feature | Function | Benefits |
---|---|---|
Context Persistence | Keeps a shared context across AI interactions | Minimizes redundant tasks and improves accuracy. |
Dynamic Scaling | Allocates resources based on system load | Boosts performance while managing resources effectively. |
State Management | Ensures synchronized states across modules | Maintains consistency during multi-step processes. |
Security Protocol | Provides encryption and access control | Safeguards sensitive data during communication. |
MCP vs. Standard APIs
Unlike standard APIs, which operate without maintaining context between requests, MCP offers a more advanced solution:
Aspect | Standard APIs | Model Context Protocol |
---|---|---|
Context Handling | Stateless operations | Built-in context persistence |
Integration Complexity | Requires multiple endpoints setup | Simplified with a single interface |
Maintenance | Manual endpoint-specific updates | Self-maintaining with automatic updates |
Scalability | Requires manual configuration | Automatically adjusts to system load |
Error Handling | Basic error responses | Advanced error recovery mechanisms |
MCP streamlines development by reducing the need for complex integration layers. Its unified interface allows developers to focus on creating AI features, rather than managing backend connections, while ensuring better system reliability and performance.
How MCP Changes AI Development
Simplifying AI Integration
MCP streamlines the process of integrating AI by offering a unified system. This eliminates the hassle of juggling different connection protocols, allowing developers to spend more time improving features. The result? A smoother path to creating tools that work well together.
Encouraging Collaboration on AI Tools
With its context-aware design, MCP makes teamwork more effective. By standardizing how components interact, it ensures that domain experts and engineers can work in sync. This alignment helps teams create AI solutions that perform reliably and meet their goals.
Leveraging MCP with Latitude
Latitude takes advantage of MCP to simplify the creation of production-ready LLMs. On the platform, domain experts focus on designing prompts while engineers manage the technical aspects. This collaboration reduces integration challenges and speeds up the development of powerful AI tools.
MCP Limits and Next Steps
Current MCP Challenges
MCP simplifies AI integration, but its broader use hinges on solving a few key technical hurdles. Many organizations still rely on outdated AI systems that aren't built for modern context-sharing protocols, making compatibility a major issue. On top of that, MCP's focus on interoperability could open up new security risks, requiring careful safeguards to protect sensitive data. Scalability is another challenge, as the protocol needs to handle larger datasets and real-time processing demands. Tackling these issues is crucial for MCP's growth and adoption. Below, we discuss practical strategies to address these obstacles.
Next Steps for AI Connection Standards
To push MCP forward, several important actions are needed:
- Updating Legacy Systems: Creating tools and frameworks to help organizations upgrade their older AI systems for better compatibility.
- Strengthening Security: Adding advanced encryption methods and strict access controls while ensuring smooth system integration.
- Optimizing Scalability: Refining algorithms and improving resource management to handle growing computational loads.
- Building Industry Standards: Collaborating with key players to develop unified protocols for seamless AI communication.
These steps are designed to solidify MCP's framework and ensure it works effectively across a variety of AI setups. By systematically addressing these challenges, organizations can unlock MCP's potential to support more collaborative AI solutions.
Conclusion
MCP represents a key development in AI system integration, acting as a universal bridge for connecting AI systems. By offering a standardized interface for AI models to interact with various data sources and tools, MCP is transforming how AI solutions are developed.
Several leading tech companies have already incorporated MCP into their systems. This highlights its practical use in simplifying integration tasks and driving new advancements. Paired with platforms like Latitude, MCP supports the creation of production-ready large language model (LLM) features while cutting down on technical complexities.
The increasing adoption of MCP underscores its ability to tackle the integration challenges previously outlined. As organizations expand their AI initiatives, MCP’s standardized framework lays the groundwork for building more advanced, interconnected systems.
With its open-source and flexible design, MCP is well-positioned for future growth, enabling AI to be applied more effectively across various industries and use cases. As the protocol evolves, it is set to become a central standard for AI interoperability, addressing current challenges while expanding its range of tools and connectors.
FAQs
How does the Model Context Protocol enhance AI performance compared to traditional APIs?
The Model Context Protocol (MCP) enhances AI performance by enabling seamless interoperability between systems. Unlike traditional APIs, MCP provides a standardized framework for sharing and managing contextual data across AI models, ensuring they can work together more effectively.
By focusing on context sharing, MCP reduces integration complexity, improves accuracy, and allows for more dynamic interactions between AI components. This makes it an essential tool for building scalable, collaborative AI systems.
What challenges might organizations face when adopting the Model Context Protocol with older AI systems?
Adopting the Model Context Protocol (MCP) with legacy AI systems can present several challenges. One common issue is compatibility - older systems may not natively support the standardized architecture of MCP, requiring significant updates or custom integrations. This can lead to increased time and resource investments during the transition process.
Another challenge is data migration. Legacy systems often rely on outdated data formats or structures, which may need to be restructured to align with MCP standards. Additionally, organizations may need to retrain staff or bring in experts to ensure seamless implementation and ongoing maintenance.
Despite these hurdles, adopting MCP offers long-term benefits, such as improved interoperability and scalability, making it a worthwhile investment for future-proofing AI infrastructure.
How does the Model Context Protocol (MCP) improve collaboration between developers and domain experts in AI projects?
The Model Context Protocol (MCP) streamlines collaboration by providing a standardized framework that bridges the gap between developers and domain experts. It ensures that AI systems can seamlessly integrate and communicate, making it easier for teams to work together on complex projects.
By offering a clear structure for defining and managing AI model contexts, MCP enables domain experts to contribute their knowledge while developers focus on technical implementation. This synergy enhances efficiency and ensures that AI solutions meet both technical and domain-specific requirements.