How To Improve LLM Factual Accuracy
Enhance the factual accuracy of large language models through quality data, fine-tuning, prompt design, and expert validation.
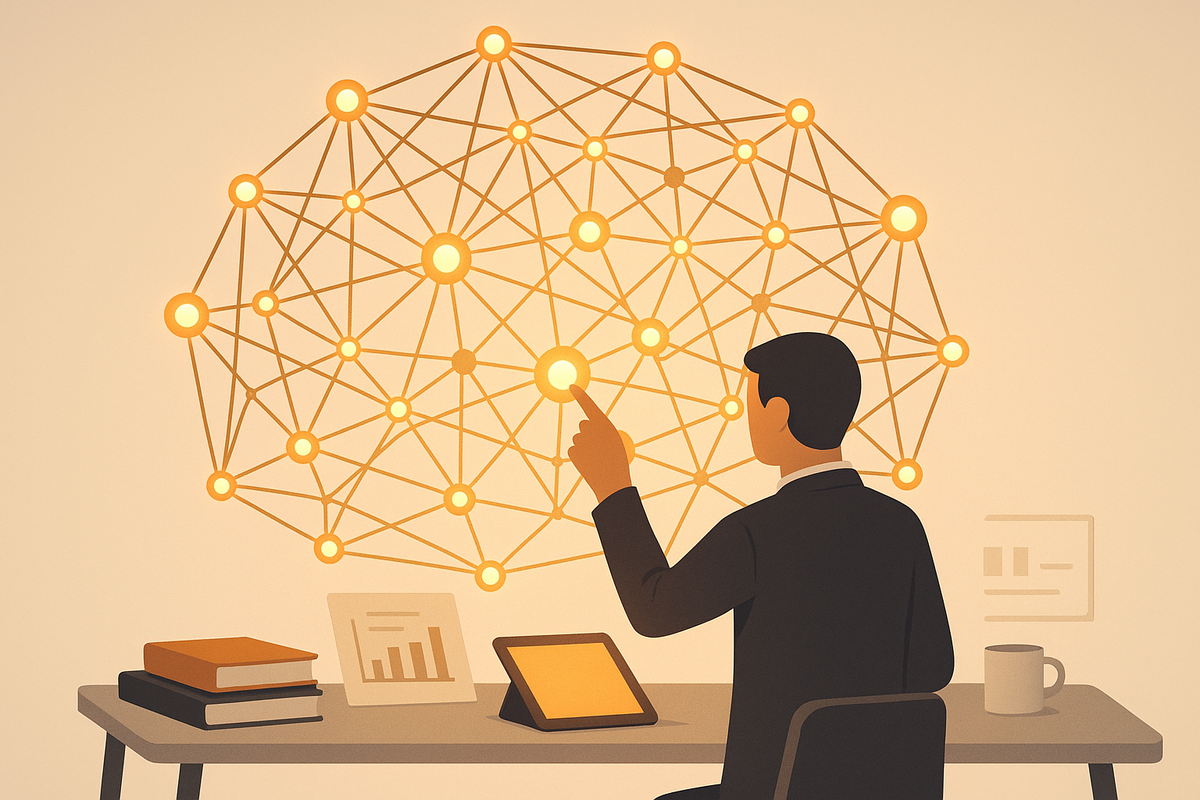
Want better accuracy from large language models (LLMs)? Start here. Improving factual accuracy in LLMs is critical for fields like healthcare, finance, and education, where mistakes can have serious consequences. Here’s how you can make LLMs more reliable:
- Use High-Quality Data: Choose verified academic sources, government publications, and expert-reviewed content.
- Fine-Tune for Specific Domains: Train models with specialized data for better precision in technical fields.
- Write Clear Prompts: Structure prompts with context, specific tasks, and output formats to guide the model effectively.
- Validate Outputs: Use expert reviews and systematic checks to verify accuracy.
These steps help reduce errors, improve reliability, and ensure safer use of AI in critical applications. Let’s dive into each method to see how they work.
Improving Training Data Quality
The quality of training data plays a crucial role in determining the accuracy of large language model (LLM) outputs. By carefully selecting reliable sources and including a mix of data types, you can reduce errors and improve model performance.
Data Source Selection
When choosing training data, prioritize these categories:
- Verified Academic Sources: Peer-reviewed journals and academic databases ensure scientifically accurate information.
- Industry Standards: Official documentation and technical specifications from trusted organizations.
- Government Publications: Reliable statistics, reports, and regulatory materials from official agencies.
- Expert-Reviewed Content: Content validated by subject matter experts to maintain accuracy.
Latitude’s collaborative platform and model training tools make it easier to validate data and integrate a variety of sources efficiently.
A well-organized validation process should include the following steps:
Validation Step | Purpose | Key Actions |
---|---|---|
Source Verification | Confirm data reliability | Check publication credentials, author expertise, and citation metrics. |
Content Review | Ensure accuracy | Cross-reference multiple sources and consult experts. |
Format Assessment | Maintain consistency | Standardize formats, clean metadata, and remove duplicates. |
Bias Detection | Address potential biases | Analyze representation and check for demographic imbalances. |
Data Source Variety
Incorporating a wide range of data sources helps LLMs gain a deeper understanding of various contexts and scenarios. Here’s how to achieve this:
Cross-Domain Integration
Merge data from related fields to provide broader context. For instance, when training an LLM for medical purposes, include:
- Clinical research papers
- Medical textbooks
- Healthcare guidelines
- Patient care protocols
- Medical terminology databases
Temporal Diversity
Include data from different time periods to reflect evolving knowledge, such as:
- Historical records
- Current research
- Emerging trends
- Updated guidelines
Format Diversity
Use a mix of content formats to enhance comprehension, such as:
- Technical documentation
- Case studies
- Research papers
- Professional guidelines
- Industry standards
Every source should meet strict validation criteria. This structured approach to data quality ensures effective fine-tuning for specific domains.
Domain-Specific Fine-Tuning
Domain-specific fine-tuning takes model accuracy to the next level by incorporating field-specific data. This process enhances the model's ability to understand and use technical terms and concepts accurately.
Industry Training Methods
The success of domain-specific fine-tuning relies on using the right training methods. Here's how to approach it effectively:
Data Preparation Phase: Organize specialized datasets based on these key criteria:
Training Component | Required Elements | Quality Indicators |
---|---|---|
Technical Vocabulary | Field-specific terms and definitions | Aligned with industry norms |
Use Cases | Practical applications and scenarios | Matches current practices |
Regulatory Content | Guidelines and compliance requirements | Verified by authorities |
Error Examples | Common mistakes and corrections | Validated by experts |
Execution Steps: Start with smaller, focused datasets and expand gradually. This allows for:
- Accurate tracking of errors
- Faster iteration cycles
- Improved quality control
- Immediate evaluation of accuracy
By combining specialized data with a structured execution plan, measurable improvements in terminology accuracy and error reduction can be achieved.
Fine-Tuning Results
This approach delivers noticeable improvements in three key areas:
- Technical Precision: Ensures the correct use of industry-specific terms.
- Contextual Relevance: Enhances the model's ability to provide accurate responses within the domain.
- Error Reduction: Reduces factual inaccuracies in outputs.
The validation process operates as a continuous cycle:
- Set baseline accuracy metrics.
- Monitor progress through iterative fine-tuning.
- Validate results with input from domain experts.
Latitude's collaborative tools allow teams to refine prompts and validate outputs throughout the process. This ensures steady improvements while meeting domain-specific needs, laying the groundwork for optimized prompt design in the next section.
Prompt Design Best Practices
Crafting effective prompts is crucial for maintaining accuracy. Well-structured prompts help reduce mistakes and improve overall precision.
Clear Prompt Writing
Writing clear prompts involves a focused approach that emphasizes specificity and context. Below are key components that enhance prompt clarity:
Component | Purpose | Example |
---|---|---|
Context Setting | Provides background and scope | "As a financial analyst reviewing Q1 2025 data..." |
Task Definition | Details specific requirements | "Calculate the year-over-year growth rate..." |
Output Format | Sets the response structure | "Present results as percentages with two decimal points" |
Constraints | Establishes boundaries and limits | "Consider only publicly reported figures" |
Latitude's collaborative tools allow teams to work together on prompt creation, ensuring accuracy and consistency across various scenarios. This systematic approach is especially helpful when dealing with complex queries.
Sequential Prompting
Breaking down complex tasks into manageable steps improves the model's performance and ensures logical reasoning throughout the process.
Follow this sequence for structured prompts:
-
Initial Data Gathering
Start by collecting and verifying basic facts. -
Intermediate Validation
Add checkpoints to confirm the accuracy of the collected data. -
Final Output Formation
Guide the model to create a concise and accurate response based on verified information.
Step Type | Purpose | Validation Method |
---|---|---|
Data Collection | Gather raw information | Cross-check with source materials |
Analysis | Process the gathered data | Use domain-specific rules |
Synthesis | Draw conclusions | Compare results against expected outcomes |
Verification | Confirm the final accuracy | Validate against established benchmarks |
Regularly review and refine prompts based on the quality of the outputs to maintain high standards.
Human Review Systems
After optimizing data and crafting precise prompts, human oversight becomes crucial for ensuring the accuracy of outputs generated by language models. This step is vital for verifying factual integrity and maintaining high-quality results.
Expert Review Process
An expert review process combines specialized knowledge with thorough validation methods. It focuses on areas where factual errors are common, ensuring outputs are both accurate and reliable.
Review Component | Purpose | Method |
---|---|---|
Domain Validation | Check technical accuracy | Specialists review outputs within their expertise. |
Factual Verification | Confirm claims and data | Compare outputs against trusted, authoritative sources. |
Consistency Check | Maintain coherent responses | Analyze multiple outputs for the same prompt to ensure uniformity. |
Edge Case Testing | Test boundary conditions | Evaluate model responses in complex or less common scenarios. |
Using tools like those from Latitude, teams can create standardized workflows for reviewers. This approach ensures consistency across various domains and helps identify recurring errors or weak points in the model's performance.
Once expert validations are complete, the next step is to apply actionable feedback to refine the model further.
Feedback Implementation
Incorporating feedback effectively requires a structured approach. Start by documenting errors, categorizing them (e.g., factual inaccuracies or contextual misunderstandings), and focusing on critical issues like high-impact mistakes or safety-related concerns.
Latitude's training tools make it easier to integrate this feedback into the refinement process, creating a cycle of continuous improvement that boosts accuracy over time.
Feedback Type | Implementation Method | Expected Outcome |
---|---|---|
Error Patterns | Adjust training data and prompts | Fewer recurring mistakes in outputs. |
Domain Gaps | Add expert knowledge | Enhanced accuracy in specialized topics. |
Context Issues | Refine prompt design | Better handling of situational nuances. |
Source Verification | Strengthen validation checks | Improved reliability in fact-checking processes. |
Latitude Platform Features
Latitude focuses on improving accuracy by refining feedback processes and fostering collaboration. As an open-source platform built for prompt engineering, it brings together domain experts and engineers to enhance the factual reliability of language model outputs. Its features build on earlier strategies, combining collaborative prompt development with precise tools for model training.
Team Prompt Development
Latitude encourages collaboration by allowing teams from different disciplines to co-create, refine, and document prompts. This setup promotes idea sharing, continuous improvement, and clear decision tracking, ensuring both technical and domain-specific knowledge are effectively integrated.
Model Training Tools
The platform includes tools designed to fine-tune models for specific domains, improving their accuracy. These tools also encourage sharing knowledge within the community, aligning with the goal of delivering better-tuned and more reliable LLM performance.
Summary and Next Steps
Improving the factual accuracy of large language models (LLMs) requires high-quality data, targeted fine-tuning, and thorough validation. This section highlights key strategies and outlines clear steps to move forward.
Here are three focus areas to enhance LLM accuracy:
- Data Quality and Fine-tuning: Continue refining data quality by applying strict criteria to data sources. Regularly review and document fine-tuning processes while conducting ongoing quality checks.
- Collaborative Prompt Engineering: Expand on earlier prompt design efforts with team-based development. Use tools like Latitude's platform for prompt testing and documentation to ensure consistent results across various applications.
- Validation Framework: Establish a validation process that combines automated testing with expert reviews. Use clear accuracy metrics and a structured feedback system to maintain high-quality outputs.
The next step is to integrate these practices into your workflows. Tools like Latitude's platform can assist with team collaboration, prompt creation, and model training to ensure reliable accuracy in production.
Start small by targeting measurable improvements, then scale these methods as you see results. Consistent application of these strategies can help maintain accuracy while expanding across different workflows.
FAQs
How can I make sure the data I use to train an LLM is accurate and trustworthy?
To ensure the data you use for training an LLM is accurate and trustworthy, focus on curating high-quality datasets. Start by collecting data from reliable sources and verify its authenticity through thorough validation processes. Golden datasets - carefully curated and labeled datasets - are particularly useful for testing and fine-tuning models.
Platforms designed for prompt engineering and collaboration, like Latitude, can help streamline this process by enabling domain experts and engineers to work together efficiently. This ensures your training data aligns with the specific needs of your LLM and maintains a high standard of quality.
What are the best practices for creating prompts that enhance the factual accuracy of LLM outputs?
To improve the factual accuracy of LLM (Large Language Model) outputs, designing effective prompts is crucial. Here are some best practices:
- Be specific and clear: Use precise instructions and include relevant context to guide the model toward accurate responses.
- Avoid ambiguity: Eliminate vague language or open-ended phrases that could lead to misinterpretation.
- Provide examples: Demonstrate the format or type of response you expect by including examples in your prompt.
By following these practices, you can significantly enhance the reliability of LLM-generated outputs. For more advanced solutions, consider tools like fine-tuning and validation methods to further refine results.
How does fine-tuning improve the accuracy of large language models for specialized domains?
Fine-tuning a large language model (LLM) for a specific domain significantly enhances its accuracy by tailoring the model to the unique terminology, context, and knowledge of that field. This process involves training the LLM on a curated dataset that reflects the specialized domain, helping it generate more contextually relevant and precise responses.
By incorporating domain-specific fine-tuning, the model becomes better equipped to understand nuanced queries, reduce factual errors, and deliver outputs that align with the expectations of experts in the field. This approach is particularly valuable in areas like healthcare, legal, or technical industries where precision is critical.