How to Build Auditing Frameworks for LLM Transparency
Learn how to establish auditing frameworks for large language models to enhance transparency, accountability, and bias mitigation in AI systems.
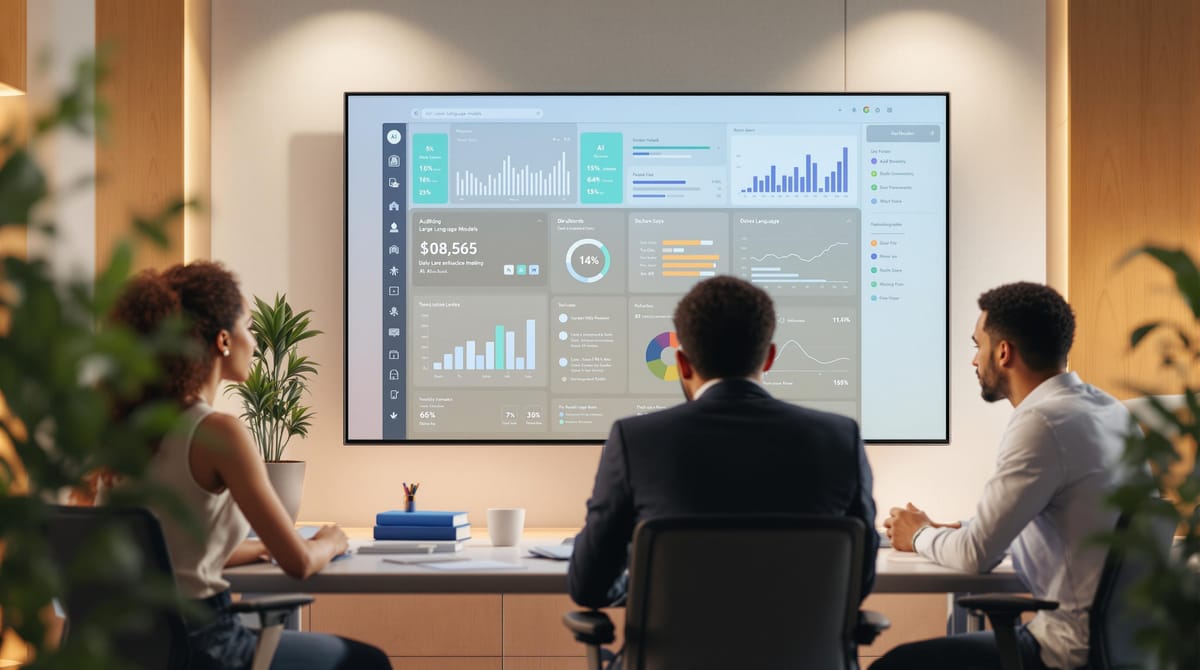
Auditing frameworks for LLMs (large language models) are essential for ensuring transparency, accountability, and reducing bias in AI systems. They help document how models are selected, tested, and deployed while meeting ethical and regulatory standards. Here’s a quick breakdown of the key steps and tools you’ll need:
Key Takeaways:
- Transparency: Keep clear records of model selection criteria, decisions, and testing outcomes.
- Accountability: Define roles, workflows, and track changes to ensure responsible management.
- Bias Mitigation: Test models for bias across demographics and address issues systematically.
5 Steps to Build an Audit Framework:
- Set Goals: Define clear objectives aligned with ethics and regulations.
- Collect Data: Document training data, outputs, and model updates.
- Design Tests: Evaluate bias, performance, and accountability with diverse scenarios.
- Run Audits: Regularly audit, document findings, and measure results against goals.
- Report: Share concise, actionable reports with stakeholders.
Tools to Streamline Audits:
- Use open-source platforms like Latitude to automate tasks, manage workflows, and document processes.
- Focus on dashboards, templates, and collaborative workspaces to simplify audits.
Addressing Common Challenges:
Challenge | Solution |
---|---|
Limited resources | Automate metrics collection and reviews. |
Data silos | Centralize data access for thorough testing. |
Inconsistent metrics | Standardize benchmarks for fairness. |
By following these steps and using the right tools, you can create an audit process that ensures your AI systems are transparent, accountable, and fair.
Core Requirements for Audit Frameworks
Audit frameworks are structured around three main pillars that ensure clarity, responsibility, and fairness:
- Transparency: Maintain clear records of model selection criteria and decision-making processes.
- Accountability: Define stakeholder roles, establish approval workflows, and implement traceable change management systems.
- Bias Mitigation: Conduct systematic bias testing across different demographic groups and put in place protocols to address any identified issues.
These pillars build upon existing documentation, metric tracking, and risk checklists to create a framework that's both thorough and practical.
Building an Audit Framework: 5 Steps
Follow these five steps to ensure clear and responsible LLM selection.
Step 1: Set Goals
Define clear audit objectives that align with ethical guidelines and legal requirements.
Step 2: Collect Data
Compile documentation on training data, output logs, and records of model updates.
Step 3: Design Tests
Create test cases to evaluate bias and accountability. Include scenarios involving sensitive content and diverse user interactions [2].
Step 4: Run Audits
Conduct audits on a regular basis. Document findings, identify patterns, and assess how results measure up against your initial goals.
Step 5: Report
Prepare concise reports for stakeholders. Combine high-level summaries with detailed analyses to provide a complete picture [3].
These steps address the need for transparency, accountability, and reducing bias, as discussed earlier.
Next, we'll look at tools designed to automate these steps and scale the auditing process.
Key question: What specific goals should be defined when setting up an audit framework for LLM transparency, and how can these goals align with ethical and legal requirements?
[2] Key question: How can organizations effectively design test cases to evaluate transparency, bias, and accountability in LLM selection processes?
[3] Key question: What are the best practices for reporting audit results to ensure clarity, accessibility, and actionable insights for stakeholders?
Audit Automation Tools
Once you've completed the design and reporting phases of an audit, automation tools can help streamline and scale each step of the process.
Open-Source Platforms for Auditing
Open-source platforms simplify LLM audits by automating tasks, managing evaluation workflows, and documenting decision-making processes. For example, Latitude offers tools for prompt engineering, collaborative features, detailed documentation, a blog, an agent library, and an engaged community. These platforms are especially useful for steps 2–4 of the audit framework.
Scaling Audit Processes
Begin with a foundational set of automated tests. Use the results to expand the scope of your audits while leaving critical decisions to expert review. Clearly document all automation rules and triggers to maintain transparency and accountability.
Making Audits Accessible
To encourage widespread use of audit processes, prioritize ease of use. Key features to focus on include:
- Dashboards that highlight essential metrics and trends
- Templates that automatically fill in audit results
- Workspaces designed to bring technical and non-technical teams together
Common Problems and Solutions
Audit teams often face practical hurdles that go beyond choosing the right tools. They need to find methods that strike a balance between accuracy, speed, and efficient use of resources.
Key Audit Challenges
- Resource limitations: Small teams and tight budgets make frequent audits difficult.
- Data silos: Fragmented data access prevents thorough bias and performance testing.
- Lack of consistent metrics: Without standardized benchmarks, evaluations can vary widely.
Effective Audit Techniques
- Cross-functional reviews: Bring together domain experts and engineers for joint sessions to uncover hidden biases.
- Continuous monitoring: Automate the collection of key metrics to quickly identify regressions.
- Blind testing: Remove model metadata from test cases to eliminate evaluator bias.
Comparing Audit Methods
Method | Advantages | Challenges |
---|---|---|
Manual audits | High precision | Time-consuming and resource-heavy |
Automated audits | Scalable and fast | Requires initial setup and integration |
These methods support the goals of transparency, accountability, and reducing bias in audits.
Conclusion
Now that you've got the tools and strategies to tackle auditing challenges, let's recap the essentials and outline your next steps.
Key Takeaways
- Develop auditing frameworks that balance technical depth with practical limitations, ensuring transparency, accountability, and a focus on reducing bias.
- Use Latitude's open-source platform to simplify documentation and make LLM testing more efficient.
Next Steps
To put the Core Requirements and five-step process into action, here’s what to do:
- Define transparency metrics that align with what your stakeholders care about most.
- Use ready-made templates to document model behavior, data sources, and test outcomes.
- Design tests that address both performance and bias-related scenarios.
- Set up a regular audit schedule and assign clear responsibilities to your team.
These steps will help you refine and expand your auditing framework as your LLM projects progress.